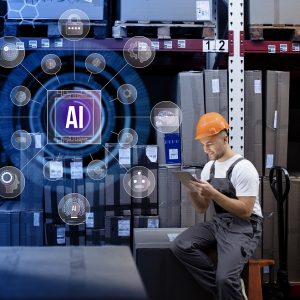
In recent years, the integration of Artificial Intelligence (AI) and Machine Learning (ML) in the cloud has revolutionized business operations. The combination of these technologies enables organizations to process vast amounts of data, derive actionable insights, and automate complex tasks. This blog explores the significance, benefits, challenges, and future of Artificial Intelligence (AI) and Machine Learning (ML) in the cloud.
The Convergence of Artificial Intelligence (AI), Machine Learning (ML), and Cloud Computing
Artificial Intelligence (AI) and Machine Learning (ML) focus on creating systems capable of performing tasks that require human intelligence. These tasks include visual perception, speech recognition, decision-making, and language translation. Meanwhile, cloud computing provides on-demand access to computing resources over the internet. This allows businesses to scale operations without significant upfront infrastructure costs.
Combining Artificial Intelligence (AI) and Machine Learning (ML) in the cloud offers several advantages:
- Scalability: Cloud platforms provide scalable resources, enabling AI and ML models to handle large datasets and extensive computations without hardware constraints.
- Cost Efficiency: Pay-as-you-go pricing models in the cloud reduce costs since businesses only pay for the resources they use.
- Accessibility: Cloud-based AI and ML services make these technologies accessible to organizations of all sizes. This eliminates the need for specialized hardware and software.
- Integration: Cloud platforms offer seamless integration with various data sources, facilitating data ingestion, processing, and analysis for AI and ML applications.
Key Cloud Platforms for Artificial Intelligence (AI) and Machine Learning (ML)
Several cloud providers offer robust Artificial Intelligence (AI) and Machine Learning (ML) services, including:
- Amazon Web Services (AWS): AWS offers a comprehensive suite of AI and ML services. These include Amazon SageMaker for building, training, and deploying ML models. AWS also provides services like Amazon Rekognition for image and video analysis and Amazon Comprehend for natural language processing.
- Google Cloud Platform (GCP): GCP provides tools like AI Platform for end-to-end ML development and deployment, and AutoML for building high-quality models with minimal effort. Google Cloud’s TensorFlow Enterprise and AI Hub are also valuable resources.
- Microsoft Azure: Azure’s AI and ML services include Azure Machine Learning for model development and deployment, Cognitive Services for pre-built AI capabilities, and the Azure Bot Service for building intelligent bots.
Benefits of Artificial Intelligence (AI) and Machine Learning (ML) in the Cloud
- Accelerated Innovation: Cloud-based AI and ML services enable rapid experimentation and iteration, allowing businesses to innovate quickly and stay competitive.
- Enhanced Decision-Making: By leveraging Artificial Intelligence (AI) and Machine Learning (ML) in the cloud, organizations can gain deeper insights from their data. This leads to more informed and strategic decision-making.
- Operational Efficiency: Automation of routine tasks and predictive maintenance using Artificial Intelligence (AI) and Machine Learning (ML) in the cloud reduces operational overhead and enhances productivity.
- Personalized Customer Experiences: AI-driven insights help businesses tailor their products and services to individual customer preferences. This improves customer satisfaction and loyalty.
Challenges of Artificial Intelligence (AI) and Machine Learning (ML) in the Cloud
Despite the benefits, integrating Artificial Intelligence (AI) and Machine Learning (ML) in the cloud presents several challenges:
- Data Privacy and Security: Ensuring the privacy and security of sensitive data in the cloud is paramount. Organizations must implement robust security measures and comply with regulatory requirements.
- Data Quality and Management: High-quality, well-labeled data is crucial for training effective AI and ML models. Managing and preprocessing large datasets can be complex and time-consuming.
- Skill Gaps: Developing and deploying AI and ML solutions require specialized skills. Organizations may face challenges in recruiting and retaining talent with the necessary expertise.
- Cost Management: While cloud services are cost-effective, managing expenses for extensive AI and ML workloads can be challenging. Organizations need to monitor usage and optimize resource allocation.
Use Cases of Artificial Intelligence (AI) and Machine Learning (ML) in the Cloud
- Healthcare: Artificial Intelligence (AI) and Machine Learning (ML) in the cloud are transforming healthcare. They enable predictive analytics, personalized medicine, and improved diagnostics. Cloud-based platforms facilitate the analysis of vast amounts of medical data, leading to better patient outcomes.
- Finance: Financial institutions use Artificial Intelligence (AI) and Machine Learning (ML) in the cloud for fraud detection, risk management, and personalized financial services. Cloud computing allows for real-time analysis and processing of large transaction datasets.
- Retail: Retailers leverage Artificial Intelligence (AI) and Machine Learning (ML) in the cloud for demand forecasting, inventory management, and personalized marketing. Cloud platforms enable the integration of data from various sources, enhancing operational efficiency.
- Manufacturing: AI-driven predictive maintenance and quality control in manufacturing improve production efficiency and reduce downtime. Cloud-based ML models analyze sensor data from industrial equipment to predict failures and optimize maintenance schedules.
Future Trends in Artificial Intelligence (AI) and Machine Learning (ML) in the Cloud
- Edge AI: The integration of AI with edge computing allows for real-time data processing closer to the source. This reduces latency and bandwidth usage, making AI applications more efficient and responsive.
- Explainable AI: As AI and ML models become more complex, there is a growing need for transparency and interpretability. Explainable AI aims to make AI decisions more understandable to humans, fostering trust and adoption.
- AI Governance: With the increasing use of AI, establishing ethical guidelines and governance frameworks is essential. Organizations must ensure that AI systems are fair, accountable, and transparent.
- Automated ML (AutoML): AutoML tools are simplifying the development of ML models. They automate tasks such as feature selection, model selection, and hyperparameter tuning. This democratizes AI and ML, enabling non-experts to build effective models.
Conclusion
The integration of Artificial Intelligence (AI) and Machine Learning (ML) in the cloud is driving significant advancements across various industries. The scalability, cost-efficiency, and accessibility of cloud platforms enable businesses to harness the power of AI and ML. This leads to improved decision-making, operational efficiency, and customer experiences. However, organizations must navigate challenges related to data privacy, quality, and skill gaps to fully realize the potential of these technologies. As Artificial Intelligence (AI) and Machine Learning (ML) in the cloud continue to evolve, trends like edge AI, explainable AI, and automated ML will shape the future of intelligent cloud computing. This paves the way for innovative solutions and transformative business outcomes.